Image Classifier
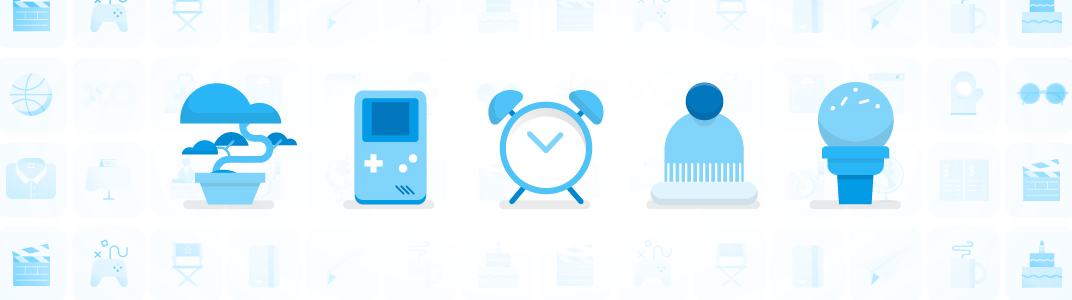
The Image Classifier demo is designed to identify 1,000 different types of objects. This demo can use either the SqueezeNet model or Google's MobileNet model architecture.
Demo: Image Classifier
This demo uses an object detection model to identify objects from an image. Try any image you like and see how accurate the model is.
You can run this demo using either the SqueezeNet model or Google's MobileNet model. Both are competitive model architectures for image recognition on mobile devices, though MobileNet generally identifies more objects from the ImageNet database accurately. Try both to see which works best for you.
What you’ll need
Assembled Vision Kit with the latest SD card image.
Step 1: Get connected
First, make sure you’re connected to your kit and have a terminal open. All the following commands must be performed on your kit's Raspberry Pi.
Step 2: Stop your current demo
Your Vision Kit might be running another demo, such as the Joy Detector, which runs by default when your kit is turned on. You can tell it's running if the green privacy LED on the front of the kit is illuminated. You can stop the Joy Detector with the following command:
sudo systemctl stop joy_detection_demo
Or if you're running another demo that uses the camera, press Control+C in the terminal to stop it.
Step 3: Find an image to use with your model
This demo requires that you pass it an image, instead of using the camera. So find an image of, well, anything! And see if the model knows what it is. You can download it with the command wget <URL>
.
For example, here's an image licensed under Creative Commons:
wget https://farm4.staticflickr.com/4110/5099896296_2e2617a0a8_o.jpg -O flower.jpg
The file is downloaded to the current directory, named flower.jpg
.
Step 4: Run the demo
Run the demo with the following command:
~/AIY-projects-python/src/examples/vision/image_classification.py --input flower.jpg
The classification results are printed in the terminal.
By default, the demo uses the MobileNet architecture. If you want to try it with SqeezeNet, specify that model as follows:
~/AIY-projects-python/src/examples/vision/image_classification.py --input flower.jpg --model squeezenet
Try both model architectures to find out which works best for your usecase.
Note: It takes a few moments for the demo to start because the raw image data must be transferred from the Raspberry Pi memory to the Vision Bonnet—the actual image classification happens very fast. This delay is avoided when processing images directly from the Pi Camera, as shown in the face_detection_camera.py
demo.
If you ran into an error, check Help.